Using Machine Learning & AI in Marketing Attribution to Elevate Campaigns
01/29/2024
The synergy between machine learning (ML) and artificial intelligence (AI) is reshaping the landscape of marketing attribution. As businesses navigate an increasingly complex web of consumer interactions across diverse channels, the integration of AI and machine learning stands out as a game-changer in deciphering the true impact of marketing efforts. This blog post delves into the intersection of these advanced technologies and marketing attribution, exploring the transformative power they bring to understanding customer journeys, optimizing campaign strategies, unlocking insights that traditional models might overlook, and more. From predictive analytics to hyper-personalization, we’ll unravel the evolving role of AI and machine learning in reshaping the future of marketing attribution.
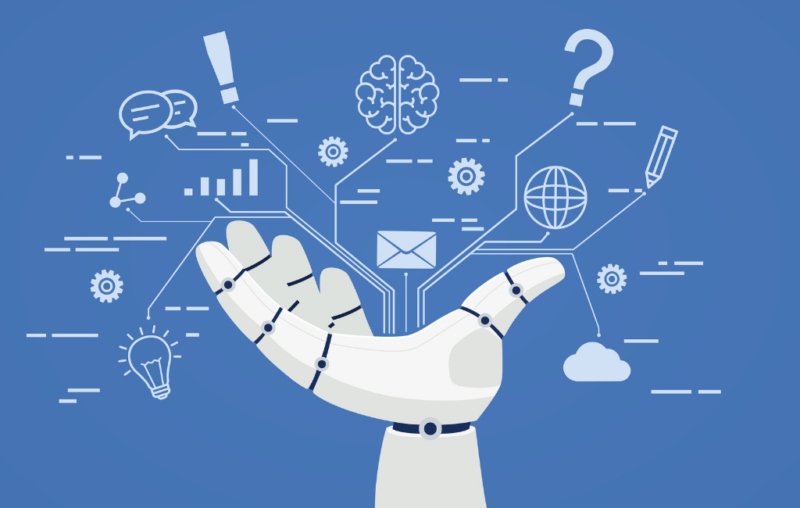
How Does Artificial Intelligence Affect Marketing?
Artificial intelligence (AI) has profoundly transformed the landscape of marketing, ushering in a new era of data-driven strategies and personalized customer experiences. One of the key ways AI impacts marketing is through enhanced data analysis and interpretation. Machine learning algorithms can process vast amounts of data, identifying non-linear relationships, patterns and trends that human marketers might overlook. This enables businesses to target their audiences more precisely, optimize advertising efforts, and streamline decision-making processes, ultimately leading to more efficient and effective marketing strategies. However, ethical considerations, privacy concerns, and the need for human oversight in AI-driven marketing remain important aspects to address as the technology continues to evolve.
Challenges with Current Marketing Attribution Models
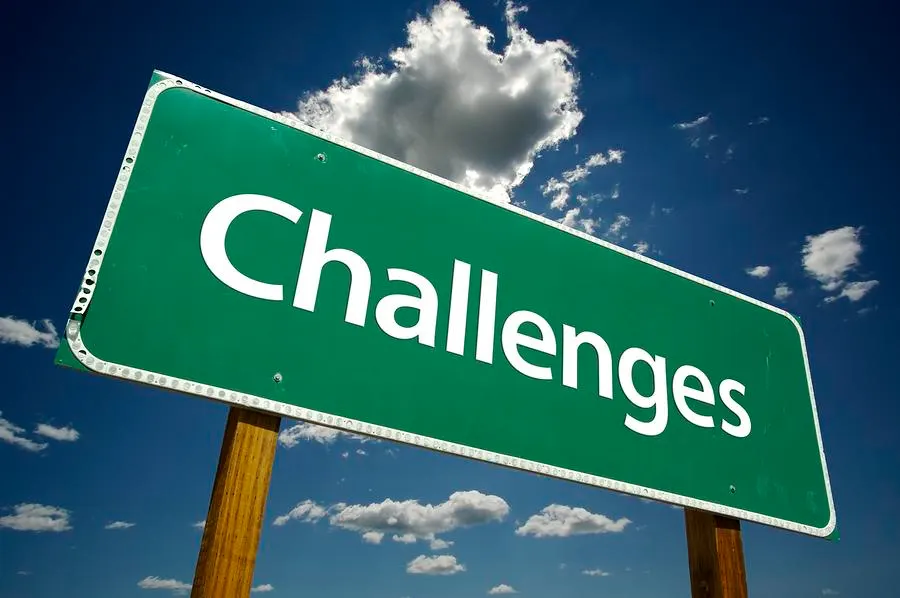
While marketing attribution models in the past played a role in understanding the effectiveness of various marketing channels and campaigns, they now present several challenges that greatly limit their accuracy, reliability and usefulness. Some of these challenges include:
Privacy Concerns
With increasing emphasis on data privacy regulations, marketers face challenges in tracking and attributing customer interactions without violating privacy standards. Striking a balance between personalization and privacy is a delicate task.
Poor Match Rates
Data loss is now so prevalent that most attribution models struggle to match even 10-20% of consumers with many brands seeing even poorer match rate performance when physical conversion points (stores, bank branches, physical locations) are in the mix. With such poor match rates, even the best 1st party databases and identity graphs struggle. The match rate issue is a major reason many brands have moved on from multi-touch attribution models.
Complex Customer Journeys
Modern customer journeys are often complex and nonlinear. Consumers interact with multiple touchpoints across various channels before making a purchase decision. Traditional attribution models may struggle to accurately assign value to each touchpoint in such intricate paths.
Cross-Device Tracking
People use multiple devices throughout their purchasing journey, making it difficult to track and attribute conversions accurately. Attribution models that don’t account for cross-device interactions may provide an incomplete picture of a customer’s journey.
Data Fragmentation
Marketers often deal with fragmented data sources, making it challenging to consolidate and integrate data from different platforms and channels. Incomplete or inconsistent data can lead to inaccurate attribution insights.
Time Lag
Attribution models may not consider the time it takes for a customer to move through the sales funnel. For instance, a customer might encounter an ad and make a purchase weeks later, and attributing the sale solely to the last touchpoint might not reflect the true impact of earlier interactions.
Attribution to Offline Channels
Many attribution models focus on online interactions, neglecting the influence of offline channels such as in-store visits, phone calls, or events. Integrating offline data into attribution models remains a challenge for many marketers.
Ad Fraud
Fraudulent activities, such as click fraud and impression fraud, can distort the performance metrics used in attribution models. Marketers need robust mechanisms to identify and filter out fraudulent data.
Benefits of AI and Machine Learning in Marketing Measurement
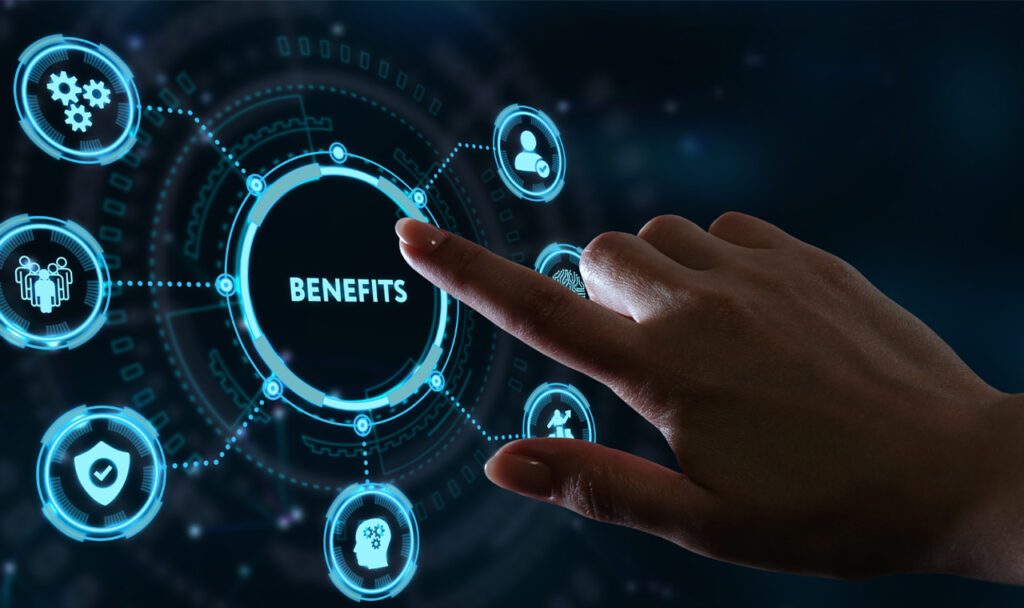
AI and machine learning bring several benefits to marketing measurement, enhancing the accuracy and efficiency of understanding the impact of various touchpoints in a customer’s journey. Some key benefits include:
Advanced Data Analysis
AI can process and analyze vast amounts of data, identifying patterns and correlations that might be too complex for traditional analytics tools. This enables a more comprehensive understanding of advertising interactions across multiple channels.
Customer Segmentation and Personalization
AI can segment customers based on their behaviors, preferences, and characteristics. This segmentation allows for more personalized marketing strategies, tailoring content and offers to specific customer segments to improve advertising relevance.
Real-Time Measurement Insights
Machine learning enables real-time analysis of data, providing marketers with up-to-the-minute insights into the performance of different channels. This agility allows for quick adjustments and optimizations to campaigns based on the most current information (“Agile” is OptiMine’s middle name).
Fraud Detection
AI algorithms can identify and mitigate ad fraud by analyzing patterns and anomalies in user behavior. This helps ensure that the data used in models is reliable and reflective of genuine customer interactions.
Automation of Repetitive Tasks
AI can automate routine tasks involved in the attribution process, freeing up time for marketers to focus on strategy and creativity. This leads to increased efficiency and allows teams to concentrate on more high-value activities.
What’s Next for Marketing and Attribution with AI and Machine Learning?
AI will inevitably impact all of our lives in one way or another, but it sometimes feels difficult to know when, where and how. In the marketing realm, early signs are showing AI’s promise across rapid-scale content development and automated testing, intelligent automation that will boost the marketing ops team’s capabilities, to fueling faster insights for brands in hyper-competitive markets. However, it is likely that brand safety will also show new strains as deep fakes and a lack of safety controls may create issues for companies who invest heavily in the quality and reputation of their brands. Privacy-centric AI must be a cornerstone – as aligning marketing strategies with evolving data privacy regulations is crucial. It seems as though the future heralds a marketing landscape where AI and machine learning will redefine the boundaries of personalization, efficiency, and strategic foresight – but the truth is, we don’t really know where all this is going, and we’re going to be really surprised along the way.
How OptiMine’s Machine Learning Can Help You
OptiMine’s solution combines machine learning, incredibly sophisticated algorithms, model automation, and cloud-based scale to deliver the most agile marketing measurement on the market. And, the best part: OptiMine’s solution is 100% privacy-safe (no identity tracking, PII, etc.), so your brand will always be in compliance with any/all privacy regulations when you work with us. Ready to learn more? Contact us today!