What is Multi-Touch Attribution?
12/07/2021
What is Multi-Touch Attribution (MTA)?
Multi-touch attribution (MTA) is a marketing measurement approach that attempts to track users across devices and the ads they’ve seen in order to determine how the ads contribute to the path to purchase. This marketing attribution approach requires user identity data and because of this, it can only be used to track addressable media (mainly digital advertising) and cannot measure traditional media such as print, TV, radio and OOH. Also, it cannot measure conversions where the consumer’s identity is not known (in-store cash transactions for example).
How Does Multi-Touch Attribution Work?
To make MTA work, a brand must align ad impressions with consumer and device identity data to determine “who saw which ad” and whether a conversion occurred. Traditionally, this relied on cookie data, but widespread browser restrictions and privacy changes have significantly diminished its viability. Now, brands must depend on third-party identity graphs along with their own first-party data to attempt to piece this information together. Further complicating this effort are major industry shifts, including Apple’s App Tracking Transparency (ATT) framework from iOS 14.5, which has drastically reduced mobile device tracking, and Google’s ongoing Privacy Sandbox initiatives. While Google has delayed the full deprecation of third-party cookies in Chrome until early 2025, features like “Tracking Protection” are already restricting cross-site tracking. Additionally, Google Topics, a key part of Privacy Sandbox, will replace individual user tracking with a cohort-based approach, eliminating consumer-level targeting for Google-owned ad networks and Chrome.
Once a consumer’s identity has been matched to an ad, a device, and a purchase transaction, the multi-touch attribution solution must then compare conversion performance of those customers who’ve seen the ad with those consumers who have not in order to determine whether the ad has generated any true lift. Compounding this complexity is that the MTA solution must also compare sequences of ads seen, keep the identity straight across multiple devices and the order of the ads seen to be able to measure the magic combination of ads that leads to the highest conversion lift. Doing this with any level of accuracy requires evaluating billions of comparisons as even a small set of campaigns across channels generates an enormous number of potential sequences and combinations. And this is fraught with accuracy problems when even a small number of conversion identities is mistakenly mis-matched with ads.
Finally, building a marketing attribution solution requires an enormous amount of data, server-to-server integrations with third parties, the exchange of PII, as well as user-ad level impression data, which is now increasingly difficult to obtain from the walled gardens. The result is typically a lengthy deployment cycle, added costs with 3rd party identity data graphs, brittle solutions that are difficult to maintain (or change), and measurement without known accuracy.
Different Types of Multi-Touch Attribution
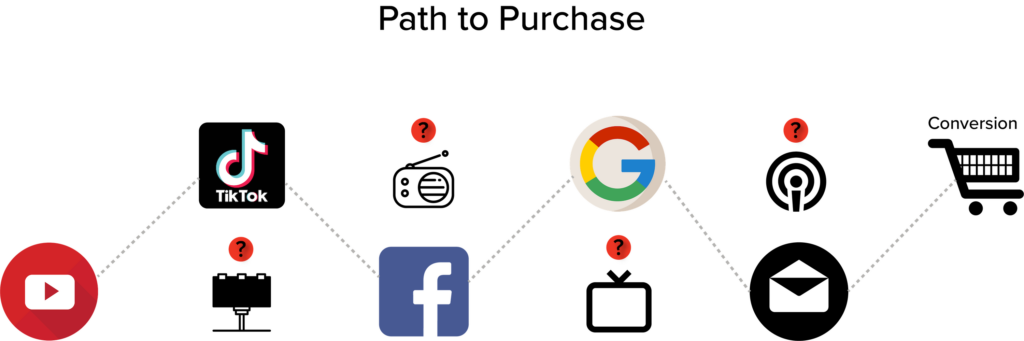
Many Multi-Touch Attribution schemes are available in the market, from very simple unsophisticated approaches to extremely complex deployments requiring heavy resources and technology. Below is an overview of the most common approaches, from simplest to most complex:
Last-Touch Attribution
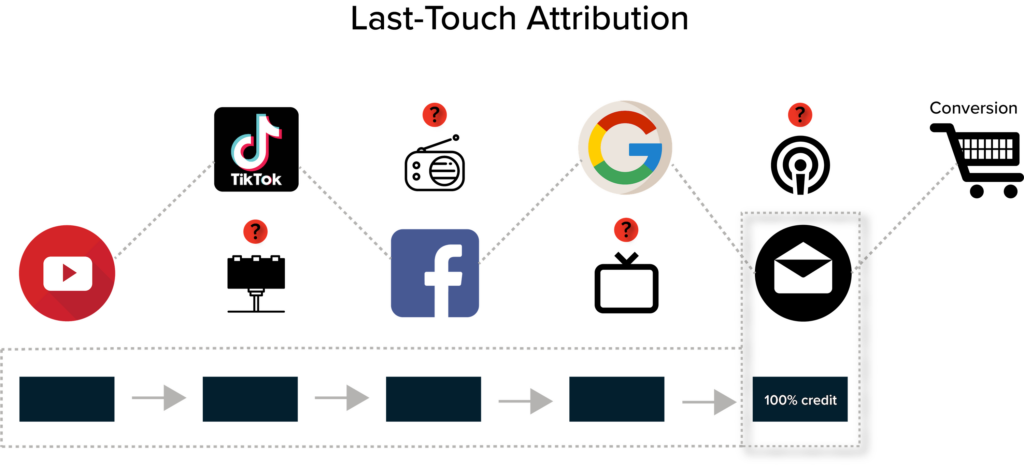
Last-Touch Attribution is the simplest form of marketing attribution. It assigns full credit for the conversion to the last ad clicked. This is the default approach of free attribution tools within Google Analytics or other web analytics solutions.
The Pros of Last-Touch Attribution:
- Last-touch is simple and easy to understand
- It doesn’t require any PII
- It is easily measured for free and doesn’t require complex software
The Cons of Last-Touch Attribution:
- It almost always over-values lower funnel ads such as paid search
- It almost always under-values all other media, especially media with low-click rates such as video, display, non-brand search, as well as any media that doesn’t have direct engagement (e.g. clicks)
- It makes a poor assumption that only clicks drive purchases, and that other media without clicks does not
- It can lead to extremely poor marketing investment decisions that will harm the brand in the long run
First-Touch Attribution
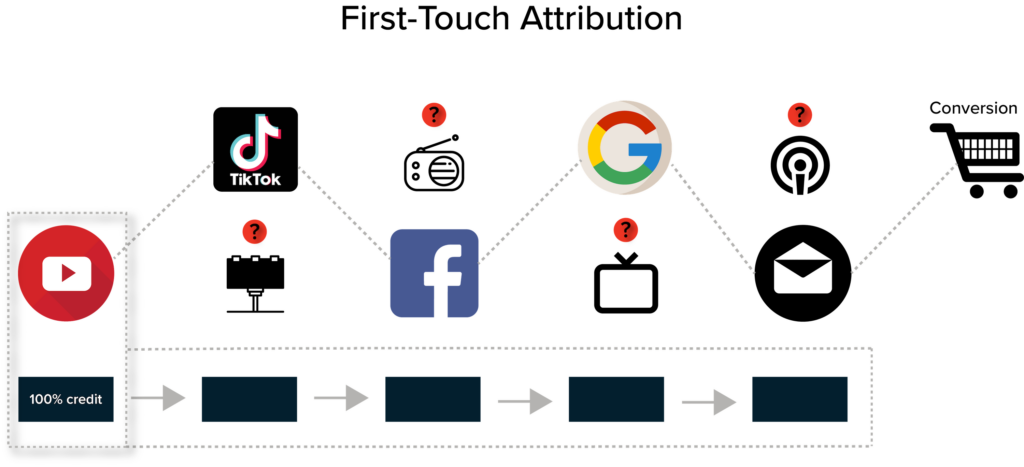
First-Touch Attribution is also a simple form of marketing attribution. It assigns full credit for the conversion to the first ad clicked. This is a common attribution option in free attribution tools within Google Analytics or other web analytics solutions.
The Pros of First-Touch Attribution:
- It gives more credit to earlier funnel media, and avoids giving all of the credit to lower funnel media
- It doesn’t require any PII
- It is easily measured for free and doesn’t require complex software
The Cons of First-Touch Attribution:
- It almost always over-values upper funnel ads that have been seen within short windows of time (1-2 weeks before conversion)
- Cookie windows and cookie expiration dramatically shorten the measurement window (and potential effects) of longer-term awareness building ads
- It relies on clicks thereby ignoring the awareness building effects of ad impressions
- It can lead to extremely poor marketing investment decisions that will harm the brand in the long run
Fractional (or Rules-Based) Attribution
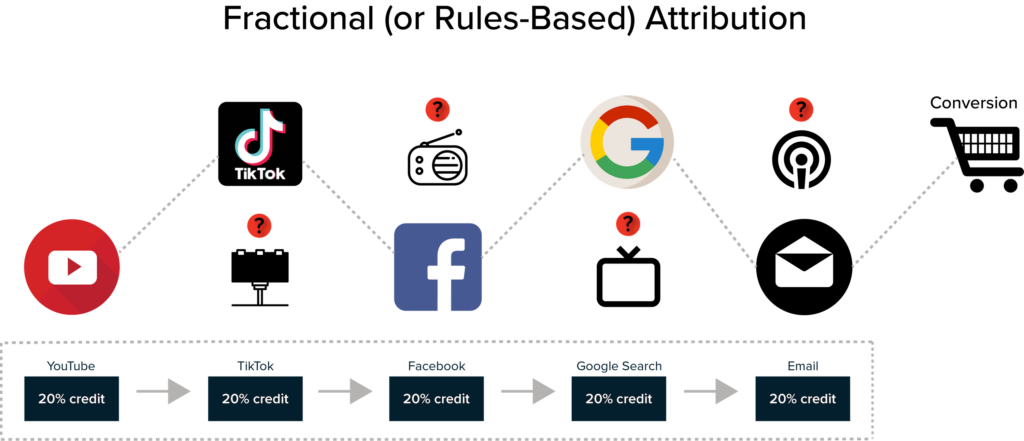
Rules-Based Attribution attempts to assign weights, factors or “rules” to give credit to different points in the funnel. While it is noble in its attempt to overcome the weaknesses of more simplistic attribution approaches such as last-touch, the weights or rules can be overly simplistic, or even just flat-out incorrect, which creates more problems than it solves.
The Pros of Rules-Based Attribution:
- Rules-Based Attribution tries to address the weaknesses of Last-Touch Attribution by giving more credit to ads that don’t usually get credit
- It can lead to more investment in upper-funnel media
- It doesn’t require any PII
- It is easily measured for free and doesn’t require complex software
The Cons of Rules-Based Attribution:
- Most of the weights are arbitrary and are not rooted in any data or evidence
- The weights are too simplistic and assign the same credit for channels that likely have significant differences among specific campaigns or ads within them
- It is usually highly inaccurate and will lead to extremely poor marketing investment decisions that will harm the brand in the long run
“U” and “W” Shaped Attribution
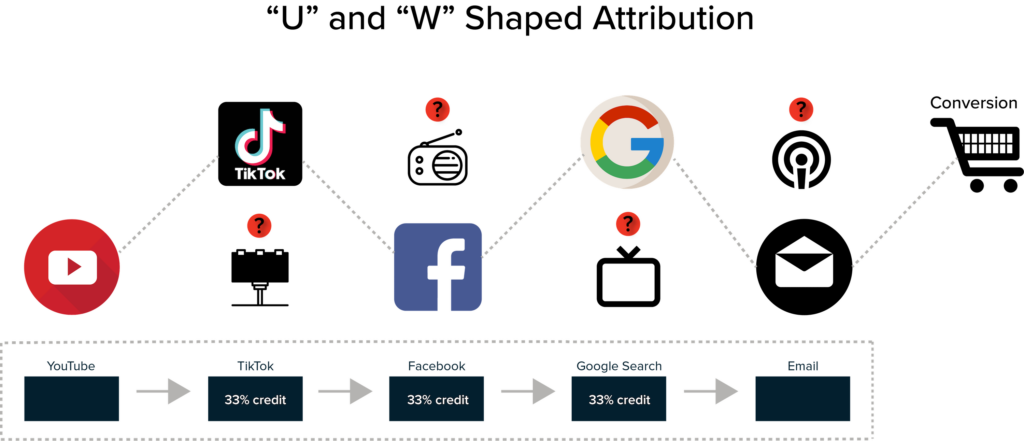
These Attribution methods also attempt to assign weights, factors or “rules” to give credit to different points in the funnel- usually by assigning more credit to the first and last ads in the sequence. In the “W”, more credit is given to the “mid-funnel” with the assumption that it contributes more too.
The Pros of “U” and “W” Shaped Attribution:
- These approaches try to address the weaknesses of Last-Touch Attribution by giving more credit to ads that don’t usually get credit
- They can lead to more investment in upper-funnel media by assuming that the first ad “seen” introduces the brand to the consumer
- They don’t require any PII
- They are easily measured for free and don’t require complex software
The Cons of “U” and “W” Shaped Attribution:
- Most of the weights are arbitrary and are not rooted in any data or evidence
- The weights are too simplistic and assign the same credit for channels that likely have significant differences among specific campaigns or ads within them
- First-touch ads are usually cut off from consideration due to cookie expiration, especially as browsers, ad platforms and mobile operating systems have moved to reduce cookies and cookie lifetimes.
- They are usually highly inaccurate and will lead to extremely poor marketing investment decisions that will harm the brand in the long run
Algorithmic Attribution
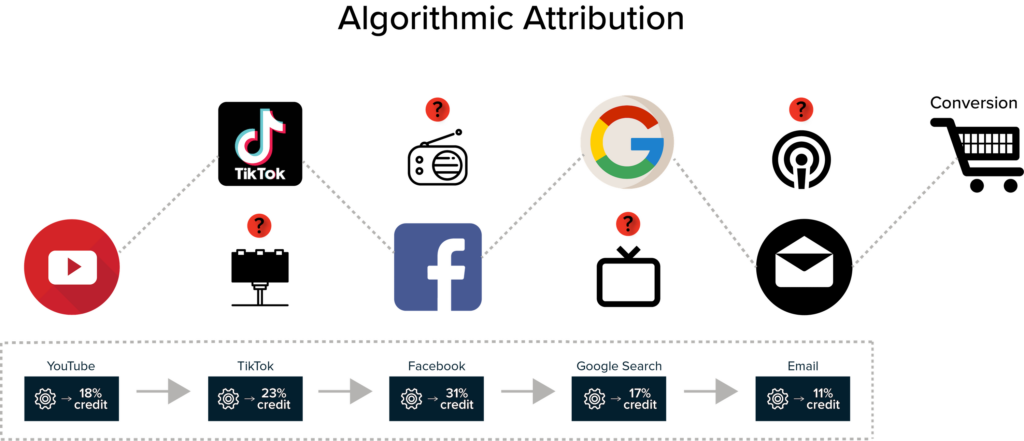
Algorithmic Attribution is a more complex approach that sometimes uses predictive models and correlations to calculate credit across ad sequences. These computations are more sophisticated than simple rules-based methods and usually require an expensive 3rd party solution to provide marketing measurement. Also, these approaches frequently move past cookie-based tracking to more complex identity-based tracking schemes.
The Pros of Algorithmic Attribution:
- These approaches try to address the weaknesses of more simplistic rules-based methods
- A model or algorithm assigns credit as opposed to simplistic methods
- These methods attempt to compare ad sequences from customers who’ve seen the ads versus those that haven’t
The Cons of Algorithmic Attribution:
- They require the use of PII and the sharing of customer data with external vendors and systems
- They suffer accuracy problems with poor customer match rates and identity mis-matches
- They require expensive software
- They ignore traditional media (unless you buy an even more expensive Marketing Mix Modeling solution add-on)
- They ignore non-marketing factors that drive conversions like promotions & discounts, the economy, the effects of the pandemic, weather, competitive factors and many other elements
- They are suffering major new gaps due to Apple iOS 14.5, Google’s upcoming FLoC initiative and other consumer data privacy moves in the market
- They are not future-proof due to the wave of privacy regulations and other tech industry changes
First-Touch Attribution vs. Multi-Touch Attribution
With a first-touch attribution model, the first marketing interaction receives 100% of the credit. While first-touch attribution only gives credit to the first marketing touchpoint, multi-touch attribution assumes that all touchpoints play some role in driving a conversion.
Last-Touch Attribution vs. Multi-Touch Attribution
Last-touch attribution is very similar to first-touch, except that the last marketing interaction is the one that receives 100% of the credit instead. While last-touch attribution only gives credit to the last marketing touchpoint, multi-touch attribution assumes that all touchpoints play some role in driving a conversion.
When to Use Multi-Touch Attribution
Given the issues around data loss, consumer tracking and privacy changes, and related model accuracy issues, what is the right role for Multi-Touch Attribution moving forward? Unfortunately, marketers who seek to understand a sequence of ads, and which sequence of ads that convert at higher rates, will be disappointed. It turns out that this is extremely difficult, complex, expensive and not all that accurate- and certainly getting more difficult and less accurate as time moves on. But for many marketers, understanding the path to purchase remains an important priority. While controlling the sequence of ads that your customers see is practically impossible, there are many qualitative reasons to examine the purchase path. Luckily, every web analytics platform provides the ability to track and visualize your customers’ purchase paths. This allows brands to understand critical information that can be used to improve performance such as:
- Path drop off rates
- Pages where customers spend more time, or places where customers get stuck and give up
- Places of potential confusion where customers reach out for support
- Different paths for consumers using mobile devices vs. desktop
- Page load times that spur drop off issues
For these Consumer Experience (CX) measures, purchase path analysis is an essential tool and measurement approach. And luckily, this doesn’t require multi-touch attribution to do so.
Data Deprecation is Causing Major Challenges for Multi-Touch Attribution
Much has been written about the consumer data privacy changes reshaping the industry, from Apple’s App Tracking Transparency (ATT) in iOS 14.5 to Google’s evolving Privacy Sandbox initiatives. Most discussions have focused on how these changes are making 1:1 ad targeting and personalization much more difficult—posing major challenges for marketers who have relied on these techniques to boost ad performance. However, an often-overlooked consequence is that these privacy changes are also severely disrupting Multi-Touch Attribution (MTA), making it far less accurate and limiting its future viability.
Consider Apple’s ATT framework, which requires apps to obtain user consent before tracking them across other apps and websites. To date, only about 15-20% of users have opted into tracking, leaving a massive data gap covering approximately 80% of iOS campaign impressions—equivalent to about 40-50% of the entire US mobile advertising market. If your MTA solution relies on tracking users across channels like Facebook, Snap, Twitter (now X), TikTok, YouTube, or Pinterest, your brand is experiencing significant data loss, leading to major new accuracy challenges.
Google is following suit. While originally planning to phase out third-party cookies in 2024, Google has now delayed the full deprecation in Chrome until early 2025. However, the rollout of Tracking Protection—a Privacy Sandbox feature that blocks cross-site tracking—has already begun affecting a subset of users. Additionally, Google Topics has replaced individual tracking with a cohort-based approach, further limiting ad personalization and attribution capabilities within the Google advertising ecosystem. These changes will create another major data gap in digital advertising, impacting not just targeting and personalization but also the accuracy of MTA solutions.
Lastly, regulatory pressure around consumer data privacy is intensifying. Several US states, including California, Virginia, Colorado, and others, have enacted privacy laws granting consumers the right to opt out of tracking, the right to be forgotten, and restrictions on data collection and resale. Non-compliance carries significant legal and financial risks. For MTA solutions—many of which rely on personally identifiable information (PII) and cross-device tracking—these new regulations introduce major challenges, forcing marketers to rethink their measurement strategies in a privacy-first world.
How to Future-Proof Marketing Attribution?
Smart brands care about properly measuring the incremental contributions of their marketing investments regardless of whether these are digital or traditional channels, and smart brands want to understand the total impact of their marketing across all conversion points- not just digital ones. But knowing that marketing attribution has become more difficult, less accurate and now has new regulatory risks, how should brands think about future-proofing their measurement? Read OptiMine’s Future-Proofing Guide for a more detailed plan of action. To get started quickly, keep these simple but effective tips in mind:
- Understand that measuring your marketing performance does not require PII nor customer/ device tracking
- Traditional methods such as Marketing Mix Modeling using modern AI, machine learning and high-speed computing provide better measurement solutions
- Understand what use cases and measurement needs match with the best tools for the job (example: tracking-based measurement is the right approach to measure consumer experience, but isn’t the right approach for marketing campaign measurement)
- Agility, speed and flexibility are required for success in a faster-moving world being disrupted by new technology-first upstarts and competitors
- Doing nothing isn’t a plan for success.
Summarizing MTA
Multi-Touch Attribution Summary:
Pros | Cons | Future-Proof Prognosis |
Good qualitative view of purchase paths and Consumer Experience (CX):
Pro Tip: these CX and purchase path measures can probably be found in your web analytics platform and don’t require additional investments in new measurement software. |
Multi-Touch Attribution doesn’t measure traditional ads:
Multi-Touch Attribution models are inaccurate (and getting worse by the day):
Marketers Can’t Control Ad Sequences:
Multi-Touch Attribution measures don’t account for non-marketing factors that drive sales, such as:
|
Poor |
Comparing OptiMine with MTA and Traditional MMM
What options are available to marketers in today’s measurement landscape, and where does OptiMine fit in? Consider your alternatives, specific needs and the options available in the market today:
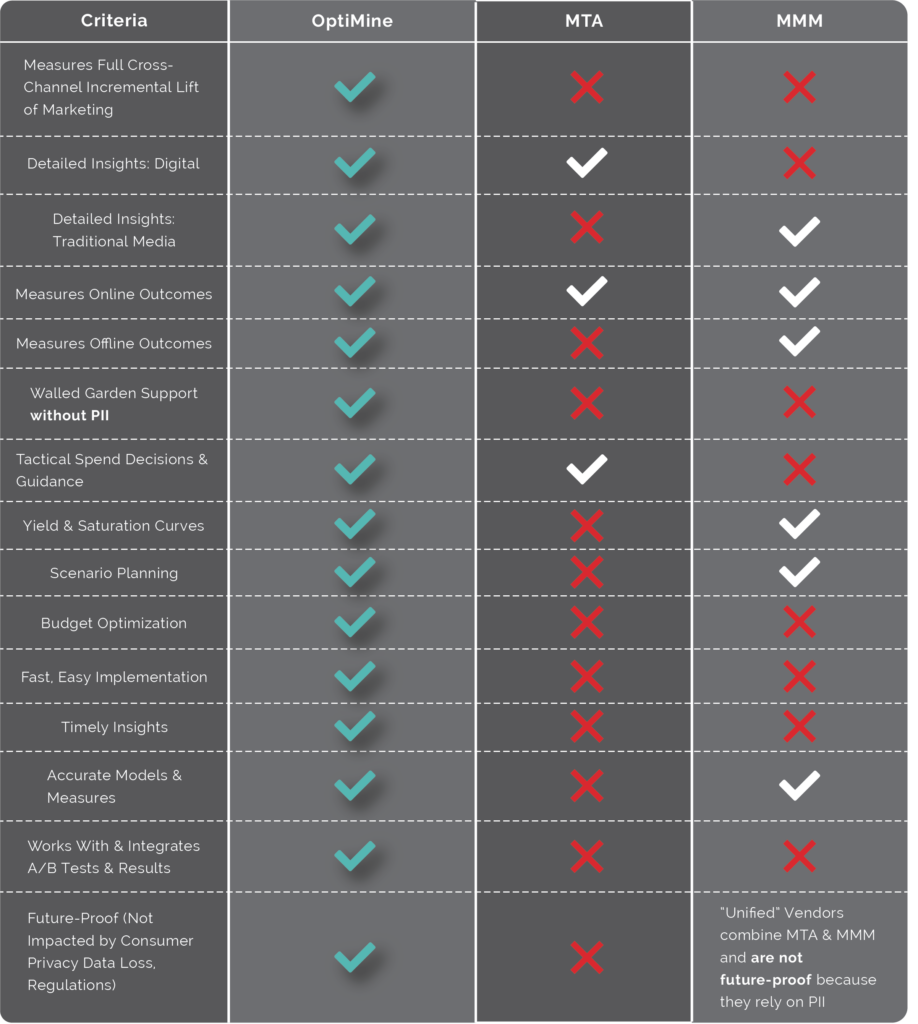
More Resources:
- “Major Consumer Privacy Changes from Apple and Google are Reshaping the Marketing & Measurement Landscapes” Blog Post
- OptiMine On-Demand Webinar: “Apple’s ATT Changes Are Now Live. Learn How It’s Impacting Brands’ Measurement, Performance, & How to Move Forward”
-
OptiMine x TickPick On-Demand Presentation: “ARF Measurement Challenges Showcase”