The Value of Incrementality Measurement in Retail Media Networks
09/27/2024
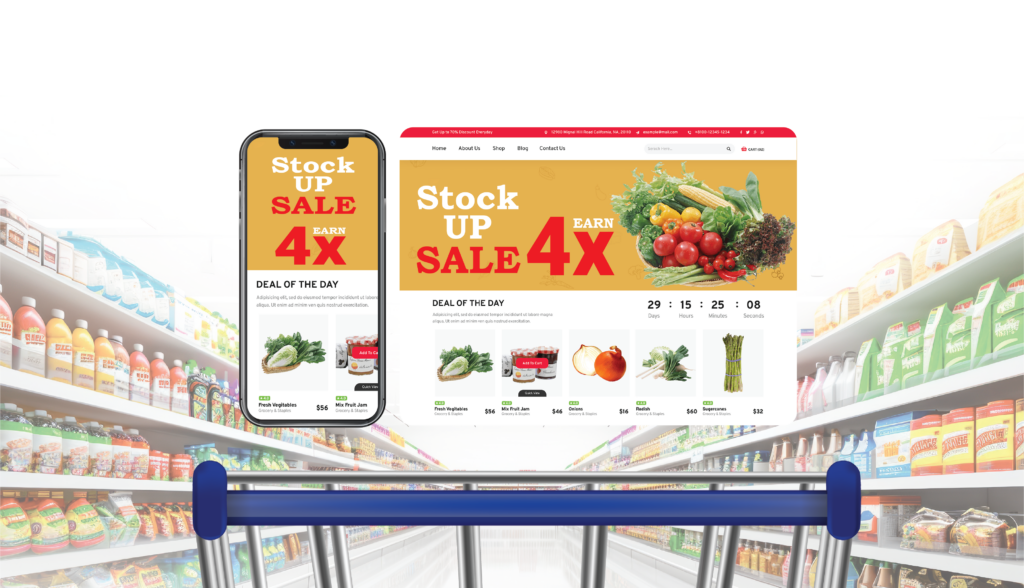
Author: OptiMine CEO, Matt Voda
Last week, I had the pleasure to co-present with a customer of OptiMine’s at the ANA’s Measurement and Analytics conference. We discussed the role of incrementality measurement in retail media networks and the topic clearly touched a nerve as we had so many audience questions that we ran out of time. There were several dozen questions that we were not able to address. While some of the audience questions were related to “how do you do incrementality measurement for retail media”, many others covered some other interesting ground:
- How do you compare measures and advertising value from one Retail Media Network to another? Is this even advisable?
- Many times, trade, promotions, operational aspects (e.g. inventory, etc.), as well as external factors such as the economy, weather, seasonality, etc. impact sales more than advertising. How do you account for these aspects?
- How do you perform incrementality measurement for in-store sales? Does this require 1st party data matching?
I’ll touch on these questions and others in a bit, but first we should begin with talking about incrementality, its use for retail media and why it is so important for brands that are trying to make sense of their (increasing) RMN investments.
Why Incrementality?
Let’s start with a premise: you are a marketer (or a marketing analytics professional) and your role is aligned with achieving a financial goal (maybe sales, profitability or something similar). With this financial goal, you want the best guidance on how to optimize your marketing investments to hit that objective. An answer that isn’t very accurate (e.g. what many RMNs provide today) doesn’t help, and in fact, could make your job a lot harder. But if you were armed with guidance that provided the true incremental impact of your advertising – even if it may show that performance to be lower in value than what is shown by other methods – your odds of success are suddenly much higher. Now, your decisions about what to do next will have a dramatically stronger impact. Queue up the promotion! This is why incrementality is so important and why it is such a problem that many marketers are not armed with it (and are not receiving it from their RMNs).
This is also why brands are demanding more from the RMNs they invest in. A BCG study from 2024 shows brands’ top request for RMN improvement is “Better Campaign Measurement”:
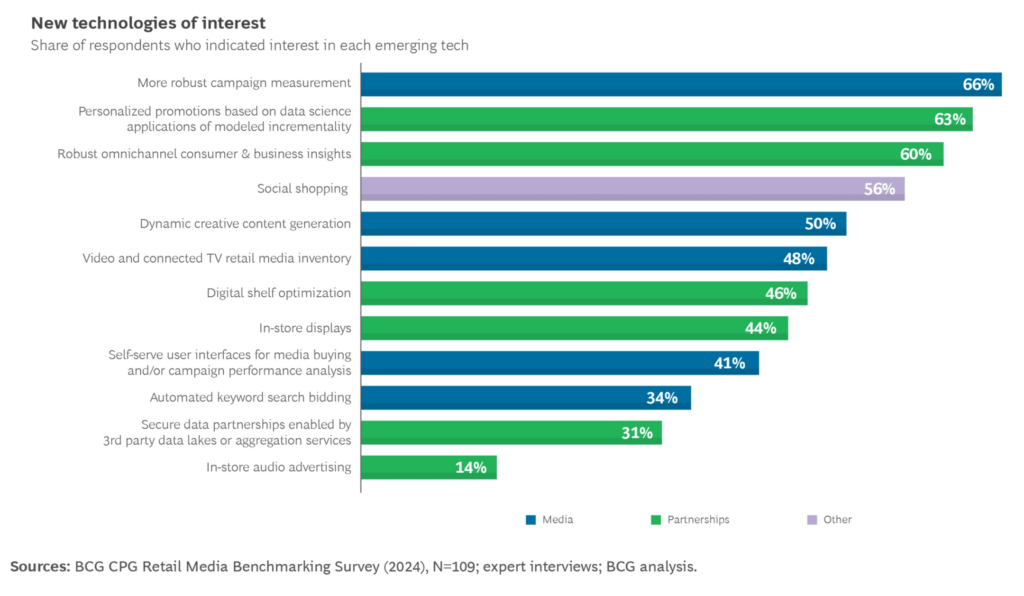
Within the collection of “Better Campaign Measurement” are brands’ requests for:
- Incrementality Measurement
- Marketing Mix Modeling Inputs
So it’s clear that brands are waking up to the importance of better measurement within RMNs. Major industry groups such as the IAB, ANA and MRC are also working to establish better measurement standards for retail media.
What is Incrementality in Retail Media?
First, it helps to look at what most RMNs are providing their advertisers today and why it is insufficient. Most RMNs are providing very basic forms of “attribution” to their advertisers and – surprise – the attribution in most cases greatly overstates the value and contributions of the advertising. Consider that most RMNs are missing true incrementality measures:
Current RMN Approach to Measurement | Resulting Challenges |
Basic Attribution: Last-Click Conversions |
If a consumer clicked on an ad and then made a purchase, the ad caused the purchase, right? Wrong. A recent Northwestern study shows that Last-Click measurement has error rates of 170%+ on average. |
Basic Attribution: View-Through Conversions |
If the ad didn’t have a click, but we know that a consumer saw an ad and then made a purchase, that un-clicked ad caused the purchase, right? Wrong.
Think about it: if your brand turned off all advertising today, you’d still sell products in the store tomorrow, the next day, and in the following weeks and months. Does this mean that advertising has zero value? No. But it does mean there are many factors that drive sales besides a brand’s ads. |
Missing Major Sales Drivers |
Sales in physical stores increase on the weekend. Likewise, sales go up during the holidays, and they also go up if there are promotions and price discounts. Shocker: a brand’s sales go down when inventory is low, or when stores close earlier. Likewise, sales go down when the economy is weaker and go up when more people have jobs.
Many RMNs do not factor in any of these aspects and simply over-attribute ads-to-sales.
Many sophisticated brands’ MMM models include these factors when measuring sales, so why is this acceptable for RMNs to be missing these? |
It should be noted that many brands equate “incrementality” with A/B testing or Randomized Control Trials (“RCTs”). While these are excellent ways to measure the incremental impact of an ad, they are practically challenging. Consider this example as a reason why: a major ad platform’s data science team counseled one of our brands that to properly test campaign impacts, the brand would need to go dark (literally, turn off the ad) for a significant portion of their target customer population for several weeks. If you believe that advertising works, you can see why this is a huge challenge in today’s high-pressure world of hitting short-term financial goals. The act of building and running a test is not hard to do (most ad platforms offer free tools to do this already) – it’s the financial risk of running the test that is difficult as well as the fact that your brand would need to do this across thousands of ads and channels to get a read on everything in the marketing budget. Not practical.
It should also be noted that many marketers assume that the way to measure incrementality is to use identity (PII) to match groups of ad-exposed customers with purchases and then compare this with cohorts of un-exposed customers with their organic purchase rates. Theoretically this is possible, and many brands have attempted this approach using multi-touch attribution approaches but even the most sophisticated brands struggle with the enormous data complexity, high IT costs, poor consumer match rates, low model accuracy and privacy risks posed by this approach.
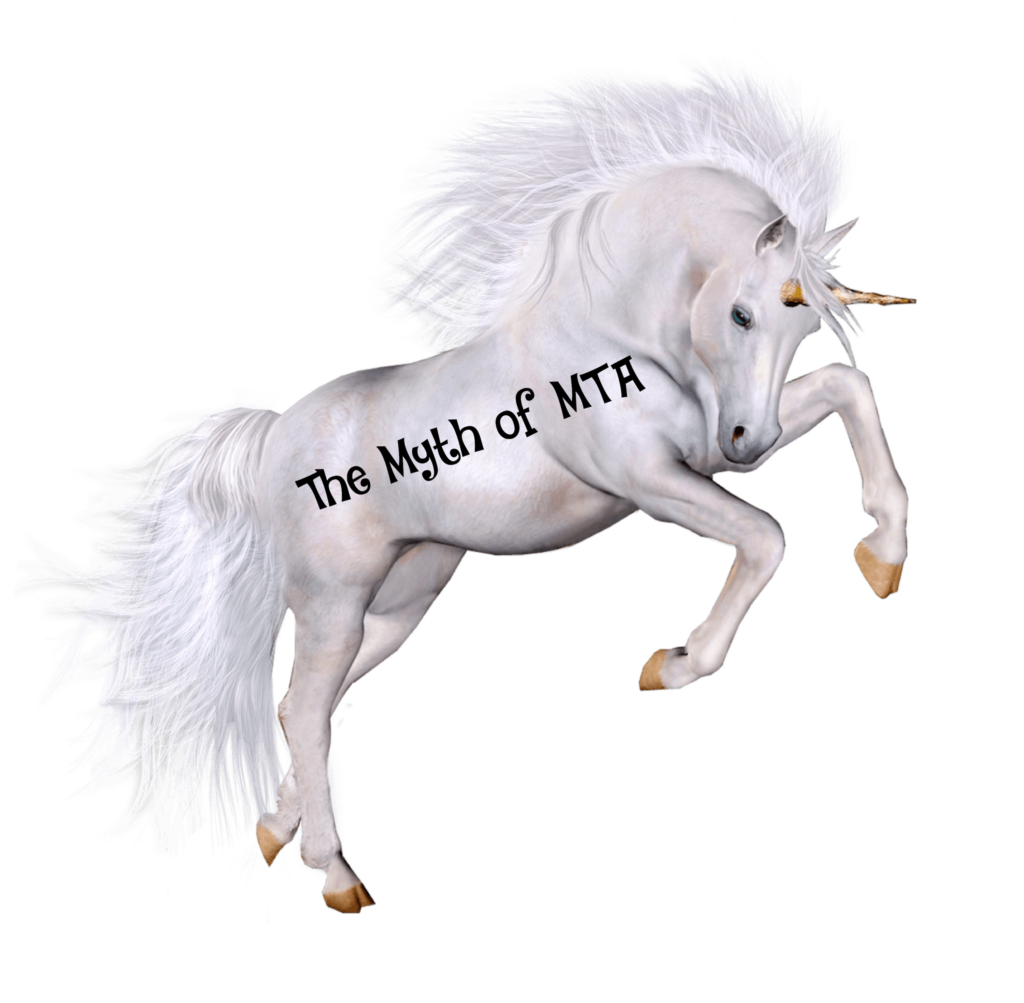
That leaves us with one potentially viable approach: marketing mix modeling (or “MMM”). MMM has gained in popularity (even though it has been used for about 40 years) because it allows a more scalable approach to measure the incremental impacts of media across the entire portfolio. The challenge for MMM is that in most forms, it isn’t very granular, which becomes a particular issue when it comes to RMNs. For a retailer operating an RMN, MMM must be able to measure thousands of advertisers, campaigns, placements and converting points. Most traditional MMMs can’t scale to meet this challenge.
For brands, there is a similar challenge. They may be operating across several RMNs, channels, placements and campaigns and traditional forms of MMM don’t scale to meeting this granular complexity.
But if the retailers and brands had the right MMM that could scale to meet this granular challenge, now the use cases shift to more optimization decisioning:
- What is the right action to take in the next week or month to hit my objective?
- How should we allocate within each RMN to drive higher performance?
- Now that we know we’re measuring the same way across all of our RMNs, how should we allocate across all RMNs in the next budget cycle to achieve our financial goals?
This is the promise of getting incrementality measurement right in the retail media space – both for retailers operating the RMNs and for the brands investing in them.
OptiMine can help. Drop us a note and we can share details about how we can answer these challenges and many more with agile marketing mix modeling. OptiMine = the Science of Success.