Marketing Mix Modeling (MMM) Explained: A Complete Guide
04/04/2024
If you’ve been watching the ad tracking and cookie demise news over the last few years, you’ll know it is becoming increasingly obvious that ad tracking is becoming more and more difficult, and that older methods for marketing measurement (such as Multi-Touch Attribution) relying on various ad tracking mechanisms are no longer useful. As time treks on, more protocols are being put into place to limit ad tracking.
Cue Google’s upcoming privacy changes: earlier this year in January, Google started formally rolling out their widely publicized shutdown of the 3rd party cookie. On January 4, 2024 Google began live testing of a Privacy Sandbox feature called “Tracking Protection” which was built to stop cross-site tracking of consumers. With this initial move, they shut down 1% of 3rd party cookies in Chrome globally, impacting nearly 30MM users giving a sense for the immense scale of the Chrome user base and the giant data loss that will follow when cookies are completely shut down (for full details of this in-market change, the Privacy Sandbox posted this update with more details and background).
Separately, Google has also communicated that they will phase out 3rd party cookies for everyone in the second half of 2024. Their latest timeline shows this will occur midway between Q3 and Q4. In the meantime, continued in-market testing will proceed and we can expect to see more public posts about the results of those tests- in particular, tests of Google’s new cohort based ad targeting approach.
Also in parallel, Google has begun notifying their largest advertisers (among which many are OptiMine clients) that many of their Google-provided performance reporting options will either be going away or will be modified significantly. Here’s a rundown of the changes:
- Path to Conversion Reporting will remain available but only for click-based paths
- Google will be relying much more heavily on their own modeled conversions (vs. observed conversions) as a result of 3rd party cookie loss (which will reduce the number of observed conversions significantly)
- Google is rolling out its own MMM toolset, although it is limited only to digital channels
These announcements and actions by Google confirm a few things that OptiMine has been communicating widely since the Privacy Sandbox was launched:
- Data and signal loss will continue and will accelerate significantly with the Google 3rd party cookie shutdown, thereby impacting Multi-Touch Attribution solutions and rendering them inaccurate
- The wider consumer privacy wave, resulting state-by-state regulations and increased risks for brands create new issues for marketers who have relied on identity for performance measurement
- Other forms of marketing measurement, including a mainstay- A/B testing- will be negatively impacted by cookie loss forcing more brands to shift to geo-based tests (which incidentally, are easy to conduct and do not require expensive software to run)
This adds up to a new imperative: brands must replace their marketing measurement approaches to avoid the issues outlined above, and must move to fundamentally privacy-safe options. User acquisition and measurement of advertising performance remains just as critical to success as it has always been—but the question now is about what form that function takes as measurement strategies shift away from consumer tracking. The answer: Marketing Mix Modeling (MMM). This type of modeling has been around for decades helping major brands measure large brand campaigns. Now, because of advanced software and automation, any brand can take advantage of this advanced analytic approach—even for mobile and in-app advertising.
What is Marketing Mix Modeling (MMM)?
Marketing Mix Modeling, also known as Media Mix Modeling— or “MMM” for short— is a statistical method for building predictive models using multivariate regression. The models evaluate media impressions and spend, non-marketing factors such as economic conditions or other elements that move business performance and converting activity over time. This is done to measure the contributions of each of these elements towards conversions (sales, traffic, customer acquisitions, engagement, branding impacts, etc.). These measures then allow the marketer to understand potential outcomes by evaluating different marketing investment levels or other scenarios.
Marketing Mix Modeling has been used by large brands for many decades and was a primary means to understand how large traditional media campaigns (TV, Print, Radio) impact sales activity. MMM can be highly accurate and is a well-proven approach to marketing measurement.
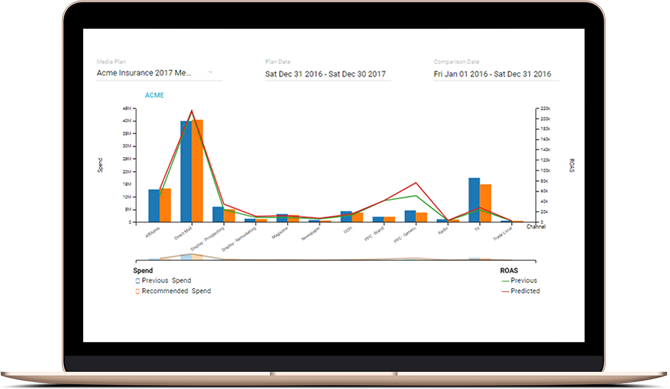
When to Use Marketing Mix Modeling
Marketing Mix Modeling is best used when a brand wants to understand and measure the incremental contributions of their marketing, and in particular, when the brand has a mix of digital and traditional marketing channels. With a more complex media mix, MMM is an ideal fit and can handle this kind of marketing complexity, and most importantly can help the brand get more performance and efficiency from their marketing budget. And with advances in AI and high-speed computing, MMM is no longer a tool just for larger global enterprises but is now an option for all brands that need advanced marketing measurement and optimization.
What Are the Components of Marketing Mix Modeling?
The components of MMM typically include:
-
Marketing Inputs or Variables: These are the elements of your marketing strategy that you want to measure and analyze. They can include advertising spend, promotions, pricing strategies, product launches, distribution efforts, and more. These are also known as the “independent variables” or “drivers” in the modeling process.
-
Sales or Performance Data: You need historical data on your sales or key performance indicators (KPIs) over a period of time. This data is crucial for understanding the relationship between your marketing inputs and outcomes. These are the “dependent variables” or “response variables” in the modeling process.
-
Data Sources: MMM relies on data from various sources, including internal data (e.g., sales data, marketing spend data), external market data (e.g., competitor data, economic indicators), and sometimes survey data or other customer feedback sources.
-
Statistical Models: Advanced statistical models are used to analyze the relationship between the marketing inputs and sales or performance data. Common modeling techniques include regression analysis, time series analysis, and machine learning algorithms. These models help identify which marketing elements have the most significant impact on outcomes.
-
Control Variables: In addition to the marketing variables, other factors that can affect sales or performance, such as seasonality, economic conditions, and industry trends, are considered. These are controlled for in the analysis to isolate the impact of marketing efforts.
-
Data Preprocessing: Data preprocessing involves cleaning, transforming, and normalizing the data to ensure that it’s suitable for modeling. This step may also involve dealing with missing data or outliers.
-
Model Validation: To ensure the accuracy and reliability of the model, it’s essential to validate it using different statistical techniques and test its ability to predict outcomes accurately. Common validation techniques include cross-validation and out-of-sample testing.
-
Insights and Recommendations: Once the model is developed and validated, it’s used to generate insights into how different marketing elements impact sales or performance. These insights are valuable for making informed marketing decisions and optimizing marketing strategies.
-
Scenario Analysis: MMM allows for scenario analysis, where you can simulate the impact of changes in marketing strategies or budgets on future sales or performance. This helps in making data-driven decisions and optimizing resource allocation.
-
Reporting and Visualization: The results of the MMM analysis are typically presented through reports and visualizations that make it easier for decision-makers to understand the findings and act on them.
-
Continuous Monitoring: Marketing Mix Modeling is an ongoing process. Marketers should continuously monitor and update their models as market conditions change and new data becomes available.
Marketing Mix Modeling Methodology
Marketing Mix Modeling (MMM) employs a systematic methodology to analyze and optimize the impact of marketing activities on business performance. The process commences with the meticulous collection of historical data on diverse marketing inputs such as advertising expenditure, pricing strategies, promotions, and product launches, which are then aligned with corresponding business outcomes like sales or revenue. Subsequently, a sophisticated statistical model is constructed, often through techniques like regression analysis or machine learning algorithms, to uncover relationships between these variables while controlling for external factors. The model parameter estimation stage involves fitting the model to historical data, followed by thorough validation to ensure its accuracy and reliability. Insights derived from the validated model allow businesses to make data-informed decisions, adjust marketing strategies, and efficiently allocate resources to maximize overall performance and profitability. This iterative process of continuous monitoring and refinement ensures that companies can adapt their marketing efforts to changing market conditions effectively.
What Are the Benefits of Modern Marketing Mix Modeling?
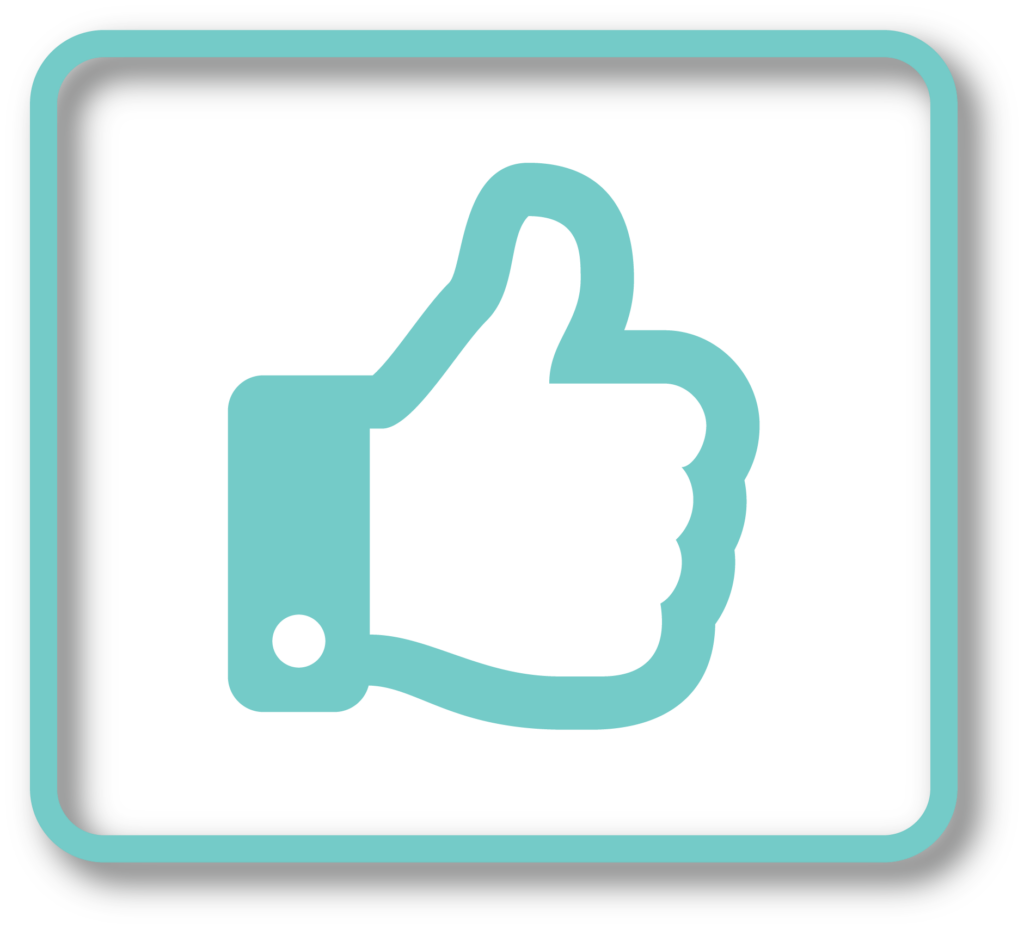
There are many advantages to using Modern MMM:
Fast.
It is built to match the speed of today’s ultra-competitive market and matches the pace of a brand’s decisions.
Agile.
Modern MMM is built for today’s complex data environment. This “data first” approach allows brands to adapt quickly, evolve the question they pose to analytics, and adjust to the constantly changing data environment.
Delivers Detailed Actionable Guidance.
With the detailed guidance that Modern MMM delivers, brands can act immediately on the data provided (unlike with Traditional Marketing Mix Modeling).
The Ability to Estimate And Measure Media Saturation and Yield Levels.
Modern MMM estimates and measures media saturation and yield levels, which allows marketers the ability to pinpoint optimal investment levels.
Scenario Planning and Budget Optimization Capabilities.
Some Modern MMM solutions also provide budget and media plan optimization capabilities. These optimization tools examine different investment levels across the entire marketing budget to create the most optimal allocation to achieve a goal or outcome. These optimization tools are beneficial because they allow marketers to make better financial decisions as well as make a case to their finance teams for specific investment approaches that will benefit the overall business.
Uses No PII.
A marketing measurement solution that won’t pose a risk to you (or your brand) due to new consumer privacy laws is crucial. Modern MMM (such as OptiMine) doesn’t use any PII, cookies, or consumer tracking EVER, making it 100% risk-free.
What are the Shortcomings of Traditional Marketing Mix Modeling?
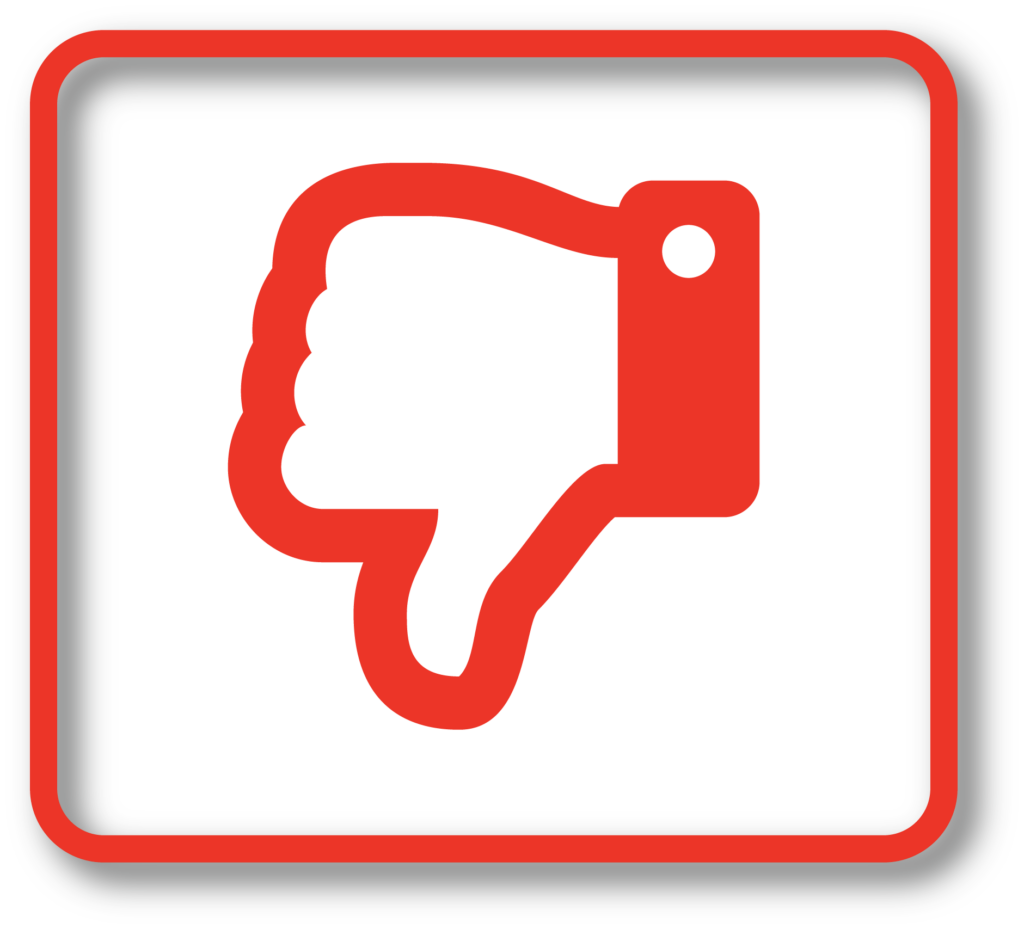
Unlike Modern MMM, Traditional Marketing Mix Modeling solutions have some significant drawbacks and considerations for brands. Most of these weaknesses are a result of the fact that the MMM models are produced and delivered by manual methods requiring expensive, deeply experienced (and expensive) consultants:
High Cost.
Traditional MMM eats up a significant percentage of marketing/analytics budget(s). There is a reason that only the largest brands in the world have used MMM in the past– they can afford to cover these significant expenses as part of their large marketing and analytics budgets.
Slow Speed.
Because Traditional MMM uses a manual consulting exercise, building models or changing them over time takes a significant amount of time. It is common for brands using Traditional Marketing Mix Modeling to get the measures many months after the period being measured.
Inflexibility.
New data, business questions, KPIs and outcomes are all part of the real-world facing marketers and brands. These factors present challenges to Traditional Media Mix Models because they all represent more manual changes, tasks, time and new costs. As a result, many deployments are highly static and don’t change much because brands don’t have the resources to evolve the models over time.
Not Actionable.
Nearly all Marketing Mix Models use highly summarized data typically rolled up by week or by month. And because the models are built using highly manual efforts, it is nearly impossible to get deeply detailed guidance from MMM measures. As such, teams get very high-level recommendations that are frequently difficult to execute with precision. This is an even bigger issue within digital marketing channels that frequently have hundreds or thousands of different campaigns, targeting elements and execution approaches, all with very different performance characteristics. These important nuances are missed completely with Traditional MMM models.
Summarizing MMM
Pros of Modern MMM | Cons of Traditional MMM |
|
|
What Are Some Examples of Marketing Mix Modeling?
For a simple example of MMM, consider the case where a brand places a billboard next to the highway. The model can now examine sales before the billboard and now whether sales have increased with the placement of the new billboard. If the brand takes the billboard down and sales drop accordingly, puts the billboard back up and sales increase again, the brand can be reasonably confident that the billboard is impacting sales positively. Well designed Marketing Mix Models also account for other factors besides media. In this case, the brand may also try to consider other factors such as the weather, promotions, seasonality, holidays and day-of-week effects before confidently concluding that the media (the billboard) is the reason for changes in sales versus these other factors that may be playing a part in sales changes over time.
While this method is probabilistic in nature, and does not attempt to match media consumption at individual customer levels using PII, it can provide a very accurate measure of the incremental contribution of marketing investments on many different types of outcomes. Plus, Marketing Mix Modeling has a major advantage over other measurement approaches such as Multi-Touch Attribution in that it can measure both digital and traditional media as well as online and offline conversion outcomes.
Here are some additional Marketing Mix Modeling examples via OptiMine case studies:
- How OptiMine helped UnitedHealthcare realize a 50x solution ROI
- How OptiMine helped Citibank uncover opportunities that other vendors missed
- How OptiMine’s Agile MMM lifted Coolibar’s online sales by 23%
- How OptiMine helped an Agency Partner deliver a 14% lift in conversions to their client
How to Future-Proof Your Marketing Measurement
Over the last 5-7 years, brands hopped on the marketing attribution bandwagon to measure digital advertising, only to be burned by poor models & measures, hidden costs, and inflexible solutions that don’t meet the needs of a modern business. Now with consumer data privacy changes hitting the market and significantly limiting the data available for Multi-Touch Attribution, brands are evaluating other options including MMM.
Unlike identity-based measurement systems, Modern Marketing Mix Modeling is not powered by user-level data. Instead, Modern MMM uses aggregated data from a range of variables and channels to examine marketing effects without tracking users. In other words, Marketing Mix Modeling doesn’t use PII and as a result, can represent a future-proof measurement option for brands. But Traditional MMM solutions and vendors can be a risk due to:
- Expensive solutions
- Slow deployments and model updates
- Guidance lacking in detail
- Inflexible solutions that are difficult to change over time
So to be fully future-proof, brands need to consider solutions and vendors that offer modern, agile solutions built for speed, scale, flexibility and precision.
Choosing Marketing Mix Modeling Software
Reference the chart below for key criteria to evaluate when choosing Marketing Mix Modeling software:
Criteria | Important Notes & Considerations |
Marketing coverage |
Does the solution cover all of the marketing channels for your brand (including traditional media such as TV, radio, print, OOH)? |
Conversion coverage |
Does the solution cover all of the conversion channels for your brand (including in-app, in-store, call center, branch locations, sales agents, in addition to e-commerce)? |
Controlling for non-marketing factors |
Does the modeling solution account and control for non-marketing factors (weather, seasonality, competition, economy) as well as your brand’s own non-marketing impacts (promotions, discounts, PR, events, sponsorships)? |
Avoiding reliance on PII |
Does the solution require PII? Can the solution provide detailed campaign-level measures without the use of PII? |
Need for speed |
Does the solution require data integrations, use of 3rd party data onboarding, tagging digital assets, matching offline consumer data, or other data-related tasks that greatly slow down deployments and updates over time? |
Flexibility first |
How easy can the solution change to new KPIs or conversion outcomes? How fast and easy is it to add new channels and data? How long must the solution recalibrate models when data changes? How long does it take to build new models? How often are the predictive models updated? |
Does the solution require additional expenses for data, data integration, web development, compliance costs, and IT costs? |
For a much more in-depth explanation on how to select the best Marketing Mix Modeling solution for your brand, read OptiMine’s “Selecting the Best Marketing Attribution Tool” blog post.
Comparing OptiMine with MTA and Traditional MMM
Criteria | OptiMine | MTA | Traditional MMM |
Measures full cross-channel incremental lift of marketing |
✓ |
X |
X |
Detailed insights: digital |
✓ |
✓ |
X |
Detailed insights: traditional media |
✓ |
X |
✓ |
Measures online outcomes |
✓ |
✓ |
✓ |
Measures offline outcomes |
✓ |
X |
✓ |
Walled garden support without PII |
✓ |
X |
X |
Tactical spend decisions & guidance |
✓ |
✓ |
X |
Yield & saturation curves |
✓ |
X |
✓ |
Scenario planning |
✓ |
X |
✓ |
Budget optimization |
✓ |
X |
X |
Fast, easy implementation |
✓ |
X |
X |
Timely insights |
✓ |
X |
X |
Accurate models & measures |
✓ |
X |
✓ |
Works with & integrates A/B tests + results |
✓ |
X |
X |
Future-proof (not impacted by consumer privacy data loss, regulations, etc.) |
✓ |
X |
“Unified” vendors combine MTA & MMM and are not future-proof because they reply on PII |
See What OptiMine Can Do for YOU!
Privacy regulations will inevitably continue to emerge, and Modern MMM provides a stable measurement tool unchanged by fluctuations in data availability, making this methodology the most resilient to privacy changes. Solve today’s marketing measurement quandaries by using a proven modern approach to Marketing Mix Modeling – OptiMine. Contact us today to learn more!
More Marketing Mix Modeling Articles:
MMM Data: What Data Do I Need for Marketing Mix Modeling?
Is Marketing Mix Modeling Still Relevant?
Attribution vs. Marketing Mix Modeling
Marketing / Media Mix Modeling FAQ
Additional Resources:
How to Measure Marketing Performance
What is ROI in Marketing and How Do You Calculate it?
Guide to Marketing Measurement
Multi-Touch Attribution is Dead
“Multi-Touch Attribution is Dead” eBook